7 different goals for your chart
A crucial step in building a powerful chart is choosing the right type of chart. A lot of charts don’t work because they simply use the wrong type of chart. To avoid this trap, we must ask ourselves a basic question: what’s the ultimate goal of our data visual? What do we want to show with our data?
Categories of graphical representation
As it turns out, there’s only a limited set of goals we might have for our chart. These goals, sometimes called ‘categories of graphical representation’, always boil down to the same seven categories.
Comparison: in many cases, we want to compare different values for different categories with each other. A bar chart is perfect to do this: the length of the bars shows us the underlying data values, and makes it easy to compare them with each other.
A part-to-whole comparison is comparison’s little brother. Rather than directly comparing categories with each other we’re comparing the size of a single category with the total size of all categories combined. Pie charts or stacked bar charts are ideal tools for this.
If our goal is to show a distribution, we want to get an impression of how data points are distributed along a certain parameter or dimension. Great charts to study or show distributions are histograms, box plots, density plots or ridgeline plots.
Correlation occurs when two parameters are related to each other. For example, if we take a large group of humans and have a look at their height and weight, we will find that, in general, taller people also have a larger weight. Typically scatter plots are used to study correlations, but there are other options as well.
Finally, hierarchy: we might want to show how different parts of a dataset are linked to each other. Maybe there’s a parent category with subcategories, and maybe those subcategories are again subdivided into even smaller categories. To show these hierarchies a sunburst diagram, treemap or network visualization might be what we need.
Start with the goal in mind
99% of all the charts we have to make fall into one of these seven categories. Clearly identifying our goal at the start of the creation process will help us find the most appropriate chart type, leading to the most powerful chart.
If you want to know more about visualizing data in the right way, you can check out the other videos in this series. Or I invite you to read my book, Powerful Charts, that will give you actionable insights and practical guidelines to create data visuals that truly engage and inspire your audience.
Full video series
- 01. Why is data visualization so powerful?
- 02. Why is data visualization so challenging?
- 03. Navigating the landscape of powerful charts
- 04. A powerful chart tells a story
- 05. A powerful chart has a high signal-to-noise ratio
- 06. Making a data visual noise-free
- 07. 7 different goals for your chart
- 08. Three roles of colour in a data visual
- 09. Choosing the right font for your data visual
- 10. Can you use Excel to create a powerful chart? (coming on January 09, 2023)
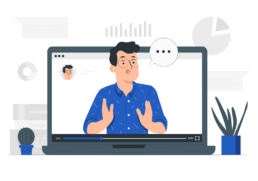
Read more:
Vreemde plaatsnamen in Vlaanderen
Iedereen kent wellicht 'Kontich' en 'Reet', maar in Vlaanderen hebben we nog veel meer merkwaardige, onverwachte, en vaak grappige plaatsnamen. Heb je bijvoorbeeld ooit al gehoord van Buitenland, Dikkebus, of Grote Homo?
9 June 2024
Small multiples can save your chart
When you're dealing with a chart that has too much information on it, the most straightforward advice to follow is: break it down into multiple charts, each with less information on them. A powerful example of this is a so-called small multiple approach.
5 September 2023
Data visualization podcasts 2023
At Baryon, we’re huge fans of podcasts! Data visualization podcasts are a great way to stay up to date on the latest trends and techniques in data visualization.
4 September 2023
Can you use Excel to create a powerful chart?
Spreadsheet tools such as Microsoft Excel or Numbers might not be the first thing on your mind when considering data visualization tools, but they can be pretty solid choices to build data visuals. Don’t let anyone convince you that using Excel to create data visuals is unprofessional.
9 January 2023
Choosing the right font for your data visual
Fonts evoke emotions: there are very sophisticated fonts, playful fonts, attention-grabbing fonts, and elegant handwritten fonts. Using the wrong type of font can have a lot of impact. In data visualization the implications of typography are mainly focused on readability. Labels and annotations can easily become so small they get hard to read. Above all else, we should choose a font which is readable at small sizes.
2 January 2023
Three roles of colour in a data visual
Colour is one of the most crucial tools we have to turn a normal chart into a powerful chart with a clear message, a chart which tells a story rather than simply presenting the information.
26 December 2022
We are really into visual communication!
Every now and then we send out a newsletter with latest work, handpicked inspirational infographics, must-read blog posts, upcoming dates for workshops and presentations, and links to useful tools and tips. Leave your email address here and we’ll add you to our mailing list of awesome people!
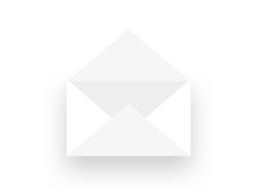